Correlation vs. Causation Worksheets
What is the difference between correlation and causation? When we are dealing with data, our operations matter extensively. In other words, mishandling or misunderstanding data can lead to disrupted manipulation. Most of data analysts misunderstood the difference between correlation and causation. They both may seem similar, but their differences can make or break a consumer-popular product. Correlation means relationship; i.e. if there is an action A and it can be related to action. On the other hand, causation explicitly means that action A causes an outcome B. In other words, the latter one is dependent on the previous one. Such is not the case for correlation as it doesn't necessarily mean that any action is dependent on the other one. Students can use these worksheets to learn how interpret if a correlation or causation can be proven or at least argued from a data set.
Aligned Standard: HSS-ID.C.9
- Growing Toddlers Step-by-step Lesson- We watch the kiddies grow and make judgments on the group.
- Guided Lesson - Measuring noise pollution with car silencers. This is actually a very big thing, worldwide.
- Guided Lesson Explanation - There is a pretty simple explanation behind these.
- Practice Worksheet - This is a real toss out of questions, some seem to be off the mark, but those are commonly found on tests. We will look at ice cream sales and the weather. You would think that weather would effect sales.
- Yes, No Worksheet - If there is a direct link between correlation and causation, let us know.
- Answer Keys - These are for all the unlocked materials above.
Homework Sheets
This type of work commands big bucks in the real world.
- Homework 1 - A survey of 70 vehicles in each of 7 cities was taken to measure the average noise pollution and the percentage of vehicles with silencers in their vehicles.
- Homework 2 - The data shows there is a positive correlation between the number of radios and people in living in a home.
- Homework 3 - There is a correlation between ice coffee and temperature. The relationship shows that, in general, the ice coffee sales increase with an increase in temperature.
Practice Worksheets
See if you think these are practical problems that for everyday students.
- Practice 1 - Which statement can be concluded about the data?
- Practice 2 - The city council has gathered data on the number of minor accidents people had. They tried to correlate the accidents to the number of years that they lived in the city.
- Practice 3 - What type of correlation does this data represent?
Math Skill Quizzes
We look for the direct link between correlation and causation.
- Quiz 1 - A company sampled production data of 15 weeks and it revealed that the average daily employee absentee rate increased with a decrease in product defects produced. Is there a direct link between correlation and causation?
- Quiz 2 - 12 randomly selected carpenters measured the amount of time they were able to make doors. Is there a direct relationship with age and stamina?
- Quiz 3 - What does the correlation show about time and the number of cities visited?
What Is a Correlation?
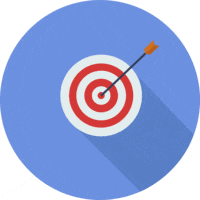
A correlation is a mathematical relationship between two numerical variables - in other words, a relationship between two statistical concepts. It is one of the five basic statistical tests and helps determine if two variables are related.
The correlation between two variables is when one process or event is affected by the other. So, for example, if you have three variables measured by one or more devices, then the association between the variables is that the devices are measured at the same time. However, correlation can be difficult to spot as it is not always visible.
If we talk about correlation in terms of analytics, it refers to the change in a variable via a change in another. The common misconception here is that the simultaneous change of the two does not necessarily have to be tied to the connection between the variables. Let's look at the three different types of correlations observed between variables:
The first one is the Positive Correlation. This type indicates that two variables are moving in the same direction, depicting a direct relation. For example, if the value of x increases, so will the value of y and vice versa.
The second one is the Negative Correlation. This type indicates that two variables are moving in the opposite direction, depicting an indirect relation. For example, if the value of x increases, the value of y will decrease and vice versa.
Lastly, we have No Correlation. This type indicates that two variables are not related to each other. For example, if the value of x increases, y either remains the same or shows no pattern.
At this point, you may have a question about how the two quantities aren't always dependent on each other, even if they show a similar pattern. This is a valid point, which we'll demonstrate with an example.
The ice cream and swimsuit sales decline during the winter season as people try to avoid cold. This depicts that sales in ice cream and swimsuits represent a negative correlation. However, the two quantities are not dependent on one another. Instead, the varying factor is the drop in temperature, which affects both quantities separately, leading to a negative correlation. This casual relationship is known as causation. Let's discuss more about causation in the following section.
What Is Causation?
In statistics, causation refers to a relationship between two or more correlated events and is an important concept to consider especially when dealing with quantitative research. It's been observed that causation is simpler to notice with the naked eye; however, it is difficult to prove or disprove a causal relationship without further analysis or research.
As a result, the topic has been labeled the most difficult and unresolved issue in statistics. Therefore, it is an important concept in statistics and attempts to identify what has caused the consequences of events. The causation considers the time in-between the variables, which means that any change in a variable will be the reason for a change in another.
Even though it's difficult to prove sometimes, without considering this, it's almost impossible to know what the data pattern means. Let's consider the same example that we used for correlation. The sales of ice cream and swimsuits didn't decline because of their relationship with one another but due to the decrease in temperature. Here, temperature shows causation as it is associated with both variables separately.
Correlation Vs. Causation
Many people confuse correlation and causation in one way or another. For example, suppose there was a correlation in the data that pre-diabetics watch a lot of television. This correlation does not imply that watching television causes pre-diabetes, nor does it imply that pre-diabetics spend an excessive amount of time watching TV.
Therefore, causation always implies correlation since variables are connected via a causal link. However, correlation does not imply causation because variables can be associated without directly impacting each other.
Reasons Why Correlation Does Not Imply Causation
Correlation and causation are important for scientific findings. For example, when our sales manager comes in after lunch, we slow down. What does the dramatic increase in sales represent if the manager doesn't come in after lunch? It will show nothing because there was a causal relationship between the two. The manager coming in after lunch correlates to increased sales, but causation can't be distinguished from coincidence. Let's look at the reasons why we can't imply causation if we have found correlation.
The use of the third variable is one of the reasons. In research, the change observed in the first two quantities is often influenced by a third variable, which indicates no cause-and-effect relationship between the two variables.
Another reason found is the chain reaction. There can be a case where different variables affect the correlation between two variables in a study. Through this chain reaction, we get to know that the two variables are correlated to each other without having a cause-and-effect relationship.
Lastly, there are some situations where we face directionality issues among variables. This means it becomes difficult to gauge which one of the two variables is dependent (effect) and the independent variable (cause). Therefore, we can't imply causation in these situations as there's no proper causal relationship between the two variables.
Real World Examples: Where Correlations Are Not Causations
This is a common phrase used in statistics. What it means is that there is not a direct cause and effect relationship between the two variables that you are studying. The purpose of most research is to determine if a direct relationship exists between two or more things. Successful research will prove that something is or is not definitely true. Often when we discuss correlations that are not causations, human error or bias are often to blame. If you stick to the data and let it guide you, you will always have the best possible outcome. The more data you have, the better picture that you will get. In the late 1950s an economist published a paper that exposed, what he thought was, a direct relationship between inflation and unemployment. It became widely accepted for just under two decades, until the 1970s hit when it just fluke based on the data he analyzed. Turns out 30 years of data is only a small segment to work with. Another thing take into account is just because the data of two variables follows a similar pattern on a graph, does not mean they are related. If similar graphs always indicated a direct relationship, the following would be true: The more married people eat margarine, the more likely they are to get a divorce. The more video games a person plays, the more likely they are to get a doctorate in Computer Science. As you can see these implications are ridiculous, but their graphs are very similar.